In my last article I discussed a number of data use cases you might want to develop in order to get the most out of your analytics investments. In my view, it's essential to prioritise and run data analytics use cases in order to stay ahead of the competition. But how can you ensure that your efforts are effective?
Successful businesses understand the importance of prioritising their data analytics projects according to their strategic business goals and objectives. By doing so, they can maximise their efficiency while minimising their costs.
Strategy is key
For those looking to get ahead of the curve, understanding how to prioritise and run data analytics use cases is key. With the right strategies in place, businesses can stay one step ahead of the competition and make decisions based on accurate information. This article will provide insight into how businesses can effectively prioritise and run data analytics use cases for maximum efficiency and effectiveness.
Definition Of A Data Analytics Use Case
A data analytics use case is simply a description of a particular process or workflow that can be used to collect, analyse and report on data. It's important to understand what each use case entails so you can prioritise them in order to effectively meet your business needs.
So, how do you define a data analytics use case?
The best way is to break it down into its business impact (based on your business objectives), the confidence in the case to deliver on the outcomes, the time it will take to implement and the way a use case can be re-used.
By recognising and understanding different data analytics use cases, you can tailor solutions that fit your organisation’s specific needs and goals. With this knowledge in hand, you can confidently move forward with creating a strategy to prioritise and run your use cases successfully. From here we can now look at how to establish goals and objectives for your business that will help inform which use cases should be prioritised first.
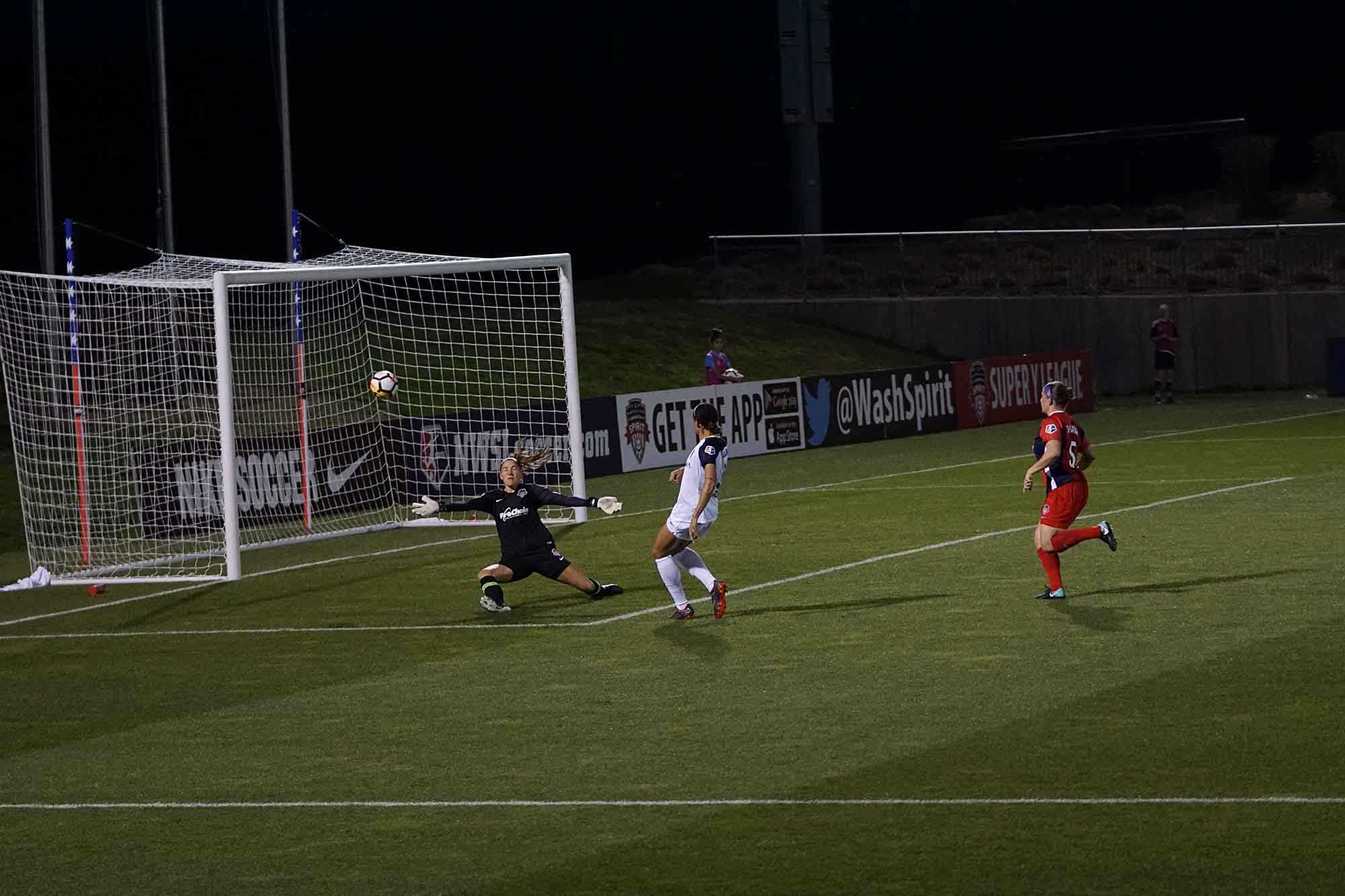
Establishing Goals And Objectives Of Your Business
It is often said that success begins with the right mindset. The same can be said for analytics use cases; having the right plan in place to prioritize them is essential. Just as a great explorer must have a map before setting out on their journey, so too must businesses have clear objectives and goals in order to choose the best data analytics use cases.
To create such a ‘map’, it is important to first establish what your specific business needs are.
Mapping with the REAN model
I use a system called REAN, which stands for reach, engage, activate and nurture. Reach might include traffic sources, engage might include how your website interacts with people, activate relates to the business goal such as a sign up, a purchase or an app download while nurture is all the ways that you get your customers to come back and consume more of what you offer.
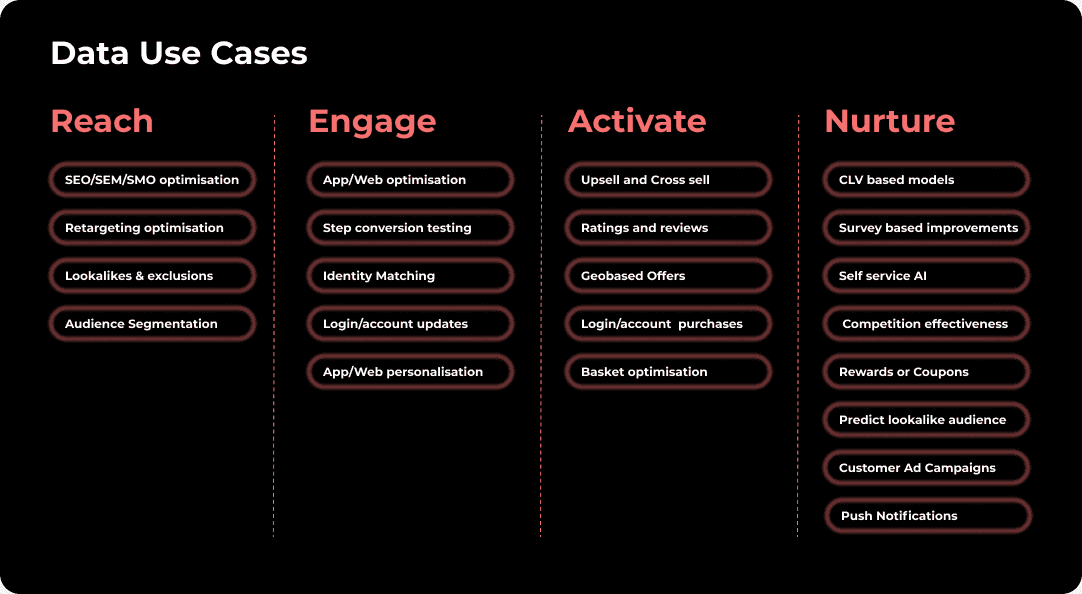
By visualising how these tasks fit together into one unified lifecycle, it becomes easier to identify which data analytics use cases should be given priority.
Having established your goals and objectives in this way, we can now move on to identifying the appropriate use cases that align with them.
Identifying Use Cases
Once you have clear objectives and goals in place, it’s time to identify the right data analytics use cases that will help you achieve them. To do this, you need to take a close look at the data available to you.
- What type of data do you have?
- What insights can be gleaned from it?
- What resources and tools will be necessary to analyse it effectively?
These are all important questions that must be answered before any use cases can be identified. Additionally, it is important to consider whether any new or additional data sources are required for the desired outcome. This could include external datasets, such as customer reviews or competitor information.
At this stage, it is also wise to think about scalability.
- What technologies and methods can you use that will allow for future growth?
- How can your existing processes be adapted or improved upon?
- Will different departments require different use cases?
Having answers to these questions will help ensure success further down the line.
With a thorough understanding of your data needs and resources, you are now in a better position to identify which analytics use cases are best suited to your organization’s objectives. Assessing business impact is the next step in bringing those ideas into reality.
Assessing Business Impact
The next step to bringing data analytics use cases to life is assessing their business impact. It’s not enough to simply identify the right use cases – they must also be able to deliver tangible results. To do this, it’s important to consider how each case will benefit your organization in terms of cost savings, increased revenue, enhanced customer experience and more.
In addition, you should examine the ease of implementation and scalability of each case.
- Will existing infrastructure and resources be sufficient?
- What technologies will need to be adopted or developed?
- Does the budget allow for any necessary investments?
- Is the expected outcome is in line with your overall goals?
Once you have a clear understanding of the potential returns on investment, you can make an informed decision about the viability of each use case.
By taking a comprehensive approach to assessing business impact, you can ensure that your data analytics projects are fruitful and effective.
Assessing Confidence In The Case
Once the business impact of each data analytics use case has been assessed, the next step is to assess confidence in the case. Is it high risk or is it very safe?
This is essential for determining whether a particular use case should be prioritised over others. To do this, it’s important to consider the accuracy and reliability of the data being used, as well as any inherent risks or challenges associated with implementation.
For example, if you are relying on third-party data sources, it’s vital that you understand their quality and identify any potential biases. You should also assess whether existing resources are sufficient to ensure successful implementation and scalability. It may be necessary to invest in additional tools or technologies if the current infrastructure does not meet your needs.
When assessing confidence in a data analytics use case, it’s important to look beyond just the technical elements. Evaluate how well aligned it is with your organisation’s business goals to ensure it will make a meaningful contribution. By taking these considerations into account, you can develop a clear understanding of which cases are most likely to succeed and should therefore be prioritised for investment.
Estimating Timeframes
At this stage, the race is on to determine how quickly each use case can be implemented. It’s time to take a deep dive into what it will take to bring the data analytics use cases to life. Estimating timeframes is a crucial part of the process, as it enables the team to develop an accurate timeline with realistic goals.
The first step is to identify any existing roadblocks that might impede progress. This could include a lack of resources or insufficient data quality. Once any potential problems have been addressed, it’s then possible to begin devising an implementation plan and estimating a timeline for completion.
It’s important to remember that no two projects are alike, and timelines can often change as more information becomes available. However, by taking into account both the technical complexity of the project and its importance in relation to organizational goals, teams can accurately predict how long it will take them to turn their data analytics use cases into reality.
Defining The Ease Of The Development Based On Timeframes
Now that the team has a better idea of how long it will take to implement each of the data analytics use cases, it’s time to refine the development process further. The goal is to determine which elements of the project require more attention and which can be accomplished fairly quickly. This allows for an organized approach to implementing the use cases and ensures that no critical steps are missed or overlooked.
Scoring the Data Use Case 1-10 For Impact, Confidence and Ease
Now that the team has identified which tasks are required to get the data analytics use cases up and running, it’s time to score them. Using a scale from 1-10, each task should be given a score based on how easy or difficult it is to complete in comparison with other tasks. For example, a 10 would indicate something relatively simple while a 1 would be assigned to something that requires more effort and planning.
This scoring system allows the team to quickly identify areas where they need to focus their energies. It also helps provide an overall picture of the work involved in setting up the data analytics use cases and gives teams insight into how best to allocate resources in order to achieve desired results.
By taking into account the scores for each use case, teams can make decisions about which elements of the project require more attention and prioritize accordingly. This organized approach ensures that critical steps are not overlooked or missed as they push forward towards success. From here, teams can move on to prioritising based on these scores.
Prioritising Based On The Score
So, the team is ready to prioritize. It’s time to decide which tasks are most important and should be given priority. As with many projects, it’s easy to get overwhelmed by the sheer volume of work required. This is why having a scoring system in place can be helpful for teams who want to ensure that their efforts are directed towards the areas that will yield the most benefit.
Simply tackle each Data Use Case (DUC) based on the score of the case. The highest score will be the one with the most impact, confidence and will be the easiest to implement. Start there.
In this way, prioritizing data analytics use cases becomes more manageable for teams and allows them to make swift progress towards success. The ability to identify which tasks are essential and focus in on those first helps teams stay organized and efficient while ensuring that nothing slips through the cracks. With clear objectives laid out, teams can now begin monitoring progress towards completion.
Monitoring Progress
With the most difficult tasks identified and tackled first, teams can now move on to monitoring progress. This step is crucial in order to ensure that all of the objectives set out for the project are being met. By keeping track of how far along each task is, teams can quickly identify any bottlenecks or roadblocks that may be preventing them from moving forward efficiently.
In addition to tracking progress, teams should also be looking for areas where they can innovate and refine their efforts. Data analytics use cases often require creative solutions and outside-the-box thinking in order to yield meaningful results. By taking a closer look at how tasks are being completed, teams can identify ways to streamline processes and improve efficiency. This will help them stay ahead of the competition and keep their organization running smoothly.
By closely monitoring progress and innovating whenever possible, teams are well positioned to take advantage of every opportunity presented to them as they strive towards success with their data analytics use cases.
Final Words
I've found that to really make the most impact, you should go for deep, narrow deployments. Identify DUCs that share the same data sources, CDP capabilities, delivery system integrations, and users. Start with setting up the system to handle the first one, and the rest of the DUCs can quickly follow suit. Build upon that foundation, adding data, features, connections, and users in small batches. Seize every opportunity that comes your way and take full advantage of the new possibilities that arise.
Shallow, broad deployments are a whole different ball game. I mean, you might have users from different departments who are all eager to jump on board with a new CDP. But trust me, serving them all right from the start is a tough nut to crack. You'd have to support countless inputs, features, outputs, and users without even getting any real value in return. So, my advice to you is to steer clear of this approach as much as possible. Stick to the focused and efficient strategy to make the most of your CDP deployment journey.
Use REAN to map the way forward, define the DUCs with that, then prioritise based on the formula above. Iterate and modify your approach as you go to be flexible but don't get sucked in to trying to please everyone all the time.
Enjoy yourself!